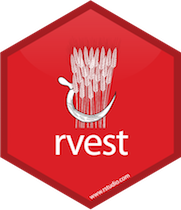
Hanjo Odendaal
www.daeconomist.com/ @UbuntR314
Rvest: https://bit.ly/2KYQZDA
Rselenium: https://bit.ly/2KWd6dW
Code Repo in Gist: https://bit.ly/2NA3Spg
Session 1:
robottxt
Rvest
Getting RSelenium
connected with docker
Session 2:
R
With great power comes great responsibility
There is a big distinction here, we have to seperate ethics vs law
Is scraping illegal?
What can I do to ensure I dont I dont expose myself or the company in a 'legal/ethical' scraping project?
I Shall Go Through Appropriate Channels if Available: If the website has a public API that provides the data, use it and avoid scraping all together
Consider The Poor Sysadmin: Provide a User Agent string that makes intentions clear and provides a way for sysadmins to contact you with questions or concerns
I Am not A Robot: Request data at a reasonable rate (~5sec wait). Don't ever be confused for a DDoS attack. Play nice with others
I shall not become addicted to data: Collecting data without a cause is very Big Brother. Only take what you need
I Shall Not Plageriase: If the website has a public API that provides the data, use it and avoid scraping all together
Not Just Interesting: Create new value from the data
Realise People Are People: Why scrape when you can just contact the holder who might SFTP it to you?
You Are Responsible, Not Your Boss: You are the expert, guide your line managers and highlight when you are uncomfortable
Web site owners use the /robots.txt file to give instructions about their site to web robots; this is called The Robots Exclusion Protocol.
User-agent: *
Disallow: /cgi-bin/
Disallow: /tmp/
Disallow: /~joe/
User-agent: Google
Disallow:
User-agent: *
Disallow: /
if(!require(robotstxt)) install.packages("robotstxt")
library(robotstxt)
rtxt <- robotstxt(domain = "www.fbi.gov")
rtxt$comments %>% tbl_df
## # A tibble: 8 x 2
## line comment
## <int> <chr>
## 1 3 # Define access-restrictions for robots/spiders
## 2 4 # http://www.robotstxt.org/wc/norobots.html
## 3 8 # By default we allow robots to access all areas of our site
## 4 9 # already accessible to anonymous users
## 5 16 # Add Googlebot-specific syntax extension to exclude forms
## 6 17 # that are repeated for each piece of content in the site
## 7 18 # the wildcard is only supported by Googlebot
## 8 19 # http://www.google.com/support/webmasters/bin/answer.py?answer=4~
FBI literally gives us almost free rein on their website!
rtxt$permissions
## field useragent value
## 1 Disallow *
## 2 Disallow * /@@search?
## 3 Disallow * /search?
## 4 Disallow * /*atct_album_view$
## 5 Disallow * /*folder_factories$
## 6 Disallow * /*folder_summary_view$
## 7 Disallow * /*login_form$
## 8 Disallow * /*mail_password_form$
## 9 Disallow * /*search_rss$
## 10 Disallow * /*sendto_form$
## 11 Disallow * /*summary_view$
## 12 Disallow * /*thumbnail_view$
## 13 Disallow * /plonejsi18n$
## 14 Disallow * /*@@castle.cms.querylisting*?
If you know exactly what you want to lookup, you can do a specific check on that url
paths_allowed("https://www.rba.gov.au/speeches")
## [1] TRUE
paths_allowed(
paths = c("/publications","/speeches"),
domain = c("https://www.rba.gov.au"),
bot = "*"
)
## [1] TRUE TRUE
Lets have a final look at the wikipedia robottxt
file
Remember as part of the commandments we must ensure our scraper behaves well and not try an access all files all at once? The crawl_delay
feature comes into play here:
rtxt$crawl_delay
## [1] field useragent value
## <0 rows> (or 0-length row.names)
But what if the robottxt
file we downloaded did not contain any information?
We need to tell R
to not go absolutely beserek and try and get all the pages we want to investigate at once, we do this using the Sys.sleep
command
nytnyt <- function (periods = c(1,1.5)){
tictoc <- runif(1, periods[1], periods[2])
cat(paste0(Sys.time()), "- Sleeping for ", round(tictoc, 2), "seconds\n")
Sys.sleep(tictoc)
}
nytnyt()
## 2018-07-06 21:21:35 - Sleeping for 1.02 seconds
Easy har-vest-ing of static websites. Welcome to rvest
if(!require(rvest)) install.packages("rvest")
library(rvest)
If you have used XML
before, rvest
is a dish of the same flavour
get_ip <- function(){
read_html("https://api.ipify.org?format=json") %>%
html_text() %>%
jsonlite::fromJSON()
}
get_ip()
## $ip
## [1] "41.2.35.15"
Now to extend on this example
ask <- read_html("http://ask.com")
ask %>% html_nodes("div")
## {xml_nodeset (15)}
## [1] <div class="l-header">\n <div class="l-header-container">\n ...
## [2] <div class="l-header-container">\n \n\n\n</div>
## [3] <div class="main">\n <div class="l-mid-content content-visible"> ...
## [4] <div class="l-mid-content content-visible">\n <div class="l- ...
## [5] <div class="l-mid-container">\n \n\n\n\n<script>\n ...
## [6] <div class="wrap-sides">\n <div class="PartialHome-wrapper" data ...
## [7] <div class="PartialHome-wrapper" data-zen="true">\n \n<div c ...
## [8] <div class="PartialHome-wrapper-logo"></div>
## [9] <div class="search-bar">\n <form accept-charset="UTF-8" ...
## [10] <div class="PartialHome-clear search-clear js-PartialHome-clear"></ ...
## [11] <div class="l-right-content content-visible">\n\n</div>
## [12] <div class="l-footer content-visible">\n <div class="l-footer-co ...
## [13] <div class="l-footer-container">\n \n\n \n\n<div class="P ...
## [14] <div class="PartialFooter-body">\n <ul class="PartialFooter-list ...
## [15] <div id="shadow" style="height: 100%; display: none;"></div>
What is the Document Object Model
html_table()
is an easy to use wrapper that identifies the nodes of class <td></td>
and <th></th>
.
Lucky for us, we don't need to know what is happening in the background! I am going to explore the rugby world Cup information from wikipedia
rugby <- read_html("https://en.wikipedia.org/wiki/Rugby_World_Cup")
rugby_tables <- rugby %>% html_table(., fill = T)
Lucky for us, we don't need to know what is happening in the background! I am going to explore the rugby world Cup information from wikipedia
rugby <- read_html("https://en.wikipedia.org/wiki/Rugby_World_Cup")
rugby_tables <- rugby %>% html_table(., fill = T)
library(scales)
rugby_tables %>%
.[[3]] %>%
mutate(attendance = as.numeric(gsub("[^0-9.-]+", "", `Total attendance`))) %>%
ggplot(., aes(Year, attendance, fill = attendance)) +
geom_bar(stat = "Identity") +
labs(title = "World Cup Rugby Attendance",
subtitle = "1987 - 2015") +
scale_y_continuous(label = comma) +
theme_light()
Ok, lets see how we can use the nodes to extract data
rugby %>%
html_nodes(., xpath = '//*[(@id = "toc")]') %>%
html_text %>%
cat
rugby %>%
html_nodes(., 'div#toc.toc') %>%
html_text %>%
cat
Read the webpage into R
pg <- read_html("https://www.ecstasydata.org/index.php?sort=DatePublishedU+desc&start=0&max=200")
pg
## {xml_document}
## <html>
## [1] <head>\n<title>EcstasyData.org: Lab Test Results for Ecstasy/Molly a ...
## [2] <body id="Home" class="TestResults">\n<div id="pre-content-top" styl ...
We will use rvest::html_table
to extract table
pg_table <- pg %>%
html_table(fill = T) %>%
.[[2]]
names(pg_table) %>% head
## [1] "Sample Photo"
## [2] "Sample NameSample Name is the street name that was submitted with the sample (or the common street-name for a table imprint or sample). The individual result view page provides more information."
## [3] "Active ContentsActive Contentsis a list of substances detected by the lab."
## [4] "Active ContentsActive Contentsis a list of substances detected by the lab."
## [5] "Date PublishedDate Published is in most cases within two weeks of the date that the tablet was received by the lab (MAPS and other non-Ecstasydata results not included)."
## [6] "Date TestedDate Tested the approximate date when the sample was tested by the lab."
pg_table <- pg %>%
html_table(fill = T) %>%
.[[2]]
name_table <- function(x){
names(x) <- c("Photo", "Name", "Substance", "Amounts",
"Date_published", "Date_tested", "Location",
"Sample_size", "Data_source")
x %>% tbl_df
}
pg_table <- pg_table %>%
name_table %>%
tbl_df %>%
slice(-1)
As with all scraping project a functional approach works amazing.
clean_table <- function(x){
# 1) Clean Amounts
# 2) Clean Locations
# 3) Coerce date
x %>%
slice(-1) %>%
select(Name, Substance, Amounts, Date_published, Location, Sample_size) %>%
filter(Substance %in% "MDMA") %>%
mutate(Amounts = ifelse(Amounts == "1" , gsub("^([0-9]+) mg.*", "\\1", Sample_size),
gsub("^([0-9]+).*", "\\1", Amounts)),
Amounts = as.numeric(Amounts)) %>%
filter(!is.na(Amounts)) %>%
mutate(Location = gsub("^(.*), .*", "\\1", Location)) %>%
mutate(Date_published = lubridate::mdy(Date_published)) %>%
select(-Sample_size)
}
We are going to build our own crawl-delay function using Sys.sleep
and runif
nytnyt <- function (periods = c(2,3)){
tictoc <- runif(1, periods[1], periods[2])
cat("Sleeping for ", round(tictoc, 2), "seconds\n")
Sys.sleep(tictoc)
}
Building the pipeline
starts <- seq(0, 2000, 200)
collect_pg <- function(x){
read_html(paste0("https://www.ecstasydata.org/index.php?sort=DatePublishedU+desc&start=", x ,
"&max=200")) %>%
html_table(fill = T) %>%
.[[2]]
}
collect_pg(starts[1]) %>%
name_table(.) %>%
clean_table(.)
## # A tibble: 65 x 5
## Name Substance Amounts Date_published Location
## <chr> <chr> <dbl> <date> <chr>
## 1 Rolls Royce MDMA 139. 2018-06-29 Zurich
## 2 Grey Duracell MDMA 250. 2018-06-24 Manchester
## 3 "Vogel\nBird" MDMA 182. 2018-06-22 Zurich
## 4 Tesla MDMA 194. 2018-06-22 Zurich
## 5 "Pik Ass\nAce of Spades" MDMA 172. 2018-06-22 Zurich
## 6 AMG MDMA 170. 2018-06-22 Zurich
## 7 Silver MDMA 194. 2018-06-22 Zurich
## 8 Philipp Plein MDMA 149. 2018-06-20 Innsbruck
## 9 "SS\nZwei S" MDMA 133. 2018-06-20 Innsbruck
## 10 Dom Pérignon MDMA 136. 2018-06-20 Vienna
## # ... with 55 more rows
A use case for a for
loop!
all_pages <- list()
for(i in 1:length(starts)){
cat("[",paste0(Sys.time()), "] Now collecting page ", i, "\n")
# Collection
all_pages[[i]] <- collect_pg(starts[i]) %>%
name_table(.) %>%
clean_table()
nytnyt()
}
all_pages %>% reduce(rbind)
Apparently, Europe is not where the strongest drugs are surfacing:
all_pages %>%
group_by(Location) %>%
summarise(Strength = mean(Amounts),
pill_count = n()) %>%
arrange(-Strength) %>%
filter(pill_count > 10) %>%
ggplot(., aes(reorder(Location, Strength), Strength, fill = Location)) +
geom_bar(stat = "Identity") +
coord_flip() +
labs(x = "Location")
Rvest is an amazing package for static website scraping and session control. For 90% of the websites out there, rvest will enable you to collect information in a well organised manner. For the other 10% you will need Selenium.
I have added extra examples features of rvest
that we will not get to today.
So onwards to Selenium!!
Once you have basic static website scraping down, you need to start learning about sessions. What does this mean?
In essence you will be simulating browser activity. Do note, its different from a browser in that it cannot render javascript, but it can simulate moving through static webpages
So what does a session object contain?
rugby <- read_html("https://en.wikipedia.org/wiki/Rugby_World_Cup")
rugby
## {xml_document}
## <html class="client-nojs" lang="en" dir="ltr">
## [1] <head>\n<meta http-equiv="Content-Type" content="text/html; charset= ...
## [2] <body class="mediawiki ltr sitedir-ltr mw-hide-empty-elt ns-0 ns-sub ...
rugby <- html_session("https://en.wikipedia.org/wiki/Rugby_World_Cup")
rugby
## <session> https://en.wikipedia.org/wiki/Rugby_World_Cup
## Status: 200
## Type: text/html; charset=UTF-8
## Size: 272641
This becomes useful when you are interacting with websites; lets take a look at forms
So to interact with forms, we are going to use html_session
and html_form
rugby <- html_session("https://en.wikipedia.org/wiki/Rugby_World_Cup")
rugby_form <- rugby %>% html_form()
rugby_form
## [[1]]
## <form> 'searchform' (GET /w/index.php)
## <input search> 'search':
## <input hidden> 'title': Special:Search
## <input submit> 'fulltext': Search
## <input submit> 'go': Go
Next, we can actually fill in the form using set_values
rugby_form[[1]]$fields$search$value
## NULL
rugby_form <- set_values(rugby_form[[1]], search = "cricket")
rugby_form
## <form> 'searchform' (GET /w/index.php)
## <input search> 'search': cricket
## <input hidden> 'title': Special:Search
## <input submit> 'fulltext': Search
## <input submit> 'go': Go
cricket <- submit_form(rugby, rugby_form)
## Submitting with 'fulltext'
cricket %>%
html_nodes("a") %>%
html_attr("href") %>%
.[10:15]
## [1] "/wiki/Cricket"
## [2] "/wiki/India_national_cricket_team"
## [3] "/wiki/The_Crickets"
## [4] "/wiki/History_of_cricket"
## [5] "/wiki/One_Day_International"
## [6] "/wiki/List_of_countries_received_ODI_status_(cricket)"